Revolutionizing Lung Cancer Diagnosis: A Comprehensive Exploration of Classification and Prediction Using Machine Learning and Deep Learning

Lung cancer remains one of the leading causes of cancer-related deaths worldwide, necessitating the development of innovative diagnostic tools to improve patient outcomes. Machine learning (ML) and deep learning (DL) techniques have emerged as powerful approaches for lung cancer classification and prediction, offering the potential to revolutionize healthcare. This article provides a comprehensive overview of the latest advancements in ML and DL for lung cancer diagnosis, empowering healthcare professionals with cutting-edge knowledge to enhance patient care.
5 out of 5
Language | : | English |
File size | : | 14709 KB |
Text-to-Speech | : | Enabled |
Screen Reader | : | Supported |
Enhanced typesetting | : | Enabled |
Print length | : | 271 pages |
Lending | : | Enabled |
Machine Learning for Lung Cancer Classification and Prediction
ML algorithms, such as support vector machines (SVMs),decision trees, and ensemble methods, have proven effective for classifying lung cancer based on various features extracted from medical images, clinical data, and genomic profiles. SVM models, in particular, have shown promising results in distinguishing malignant lung nodules from benign ones, aiding in early detection and guiding treatment decisions.
Deep Learning for Lung Cancer Classification and Prediction
DL, a subset of ML, has gained significant attention in recent years due to its exceptional performance in complex pattern recognition tasks. Convolutional neural networks (CNNs),a type of DL architecture, have demonstrated remarkable accuracy in classifying lung cancer subtypes, including adenocarcinoma, squamous cell carcinoma, and large cell carcinoma, from CT images.
Clinical Applications of ML and DL in Lung Cancer
The integration of ML and DL into clinical practice has opened new avenues for lung cancer diagnosis and management. These techniques have been employed in:
- Early detection: Screening high-risk populations using ML and DL algorithms to identify suspicious lung nodules at an early stage, increasing the chances of successful treatment.
- Accurate diagnosis: Assisting pathologists in classifying lung cancer subtypes based on histopathology images, leading to more precise treatment planning and improved patient outcomes.
- Personalized treatment: Predicting the response to specific therapies using ML and DL models based on individual patient characteristics, enabling personalized treatment approaches.
- Prognostication: Assessing the likelihood of recurrence and survival using ML and DL algorithms, providing valuable information for patient counseling and follow-up care.
Challenges and Future Directions
While ML and DL have shown great promise in lung cancer diagnosis, several challenges remain:
- Data availability and quality: Accessing large, high-quality datasets is crucial for training robust ML and DL models.
- Interpretability: Understanding the decision-making process of ML and DL models is essential for building trust among healthcare professionals and patients.
- Integration into clinical workflow: Seamlessly integrating ML and DL tools into clinical practice to ensure efficient and effective adoption.
Ongoing research efforts are addressing these challenges, and future advancements in ML and DL hold the potential to further revolutionize lung cancer diagnosis and treatment.
The application of ML and DL techniques in lung cancer classification and prediction has transformed the field of healthcare, providing clinicians with powerful tools to improve patient care. These techniques enhance early detection, facilitate accurate diagnosis, guide personalized treatment decisions, and improve prognostication. As the field continues to evolve, ML and DL will undoubtedly play an increasingly vital role in the fight against lung cancer, leading to better outcomes for patients worldwide.
Learn more about our cutting-edge ML and DL solutions for lung cancer diagnosis.
5 out of 5
Language | : | English |
File size | : | 14709 KB |
Text-to-Speech | : | Enabled |
Screen Reader | : | Supported |
Enhanced typesetting | : | Enabled |
Print length | : | 271 pages |
Lending | : | Enabled |
Do you want to contribute by writing guest posts on this blog?
Please contact us and send us a resume of previous articles that you have written.
Book
Novel
Page
Chapter
Text
Story
Genre
Reader
Library
Paperback
E-book
Magazine
Newspaper
Paragraph
Sentence
Bookmark
Shelf
Glossary
Bibliography
Foreword
Preface
Synopsis
Annotation
Footnote
Manuscript
Scroll
Codex
Tome
Bestseller
Classics
Library card
Narrative
Biography
Autobiography
Memoir
Reference
Encyclopedia
Iris Chang
Jackson A Thomas
Ian Osborn
Scott Klusendorf
Iona Holloway
Hugh Sebag Montefiore
Karen Grigsby Bates
Jack D Forbes
Ibbie Ledford
Jackie Jouret
Iain Jackson
Hugh Thompson
Jacinta Lu Costello
Iain Borden
Howard Zinn
S K Dewan
Steve Dunwell
Jaime A Pineda
Lorraine Stutzman Amstutz
J David Markham
Light bulbAdvertise smarter! Our strategic ad space ensures maximum exposure. Reserve your spot today!
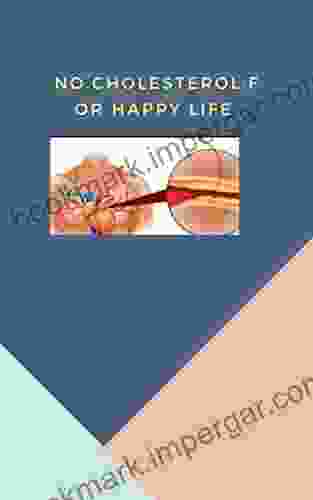

- Hugo CoxFollow ·10.2k
- Harrison BlairFollow ·13.5k
- Chinua AchebeFollow ·19.5k
- Chandler WardFollow ·19.5k
- Emanuel BellFollow ·2.9k
- Winston HayesFollow ·15.8k
- Bruce SnyderFollow ·8.1k
- J.R.R. TolkienFollow ·10.8k
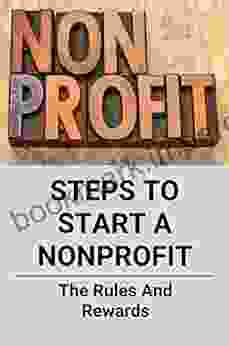

Unlock Your Nonprofit Potential: A Comprehensive Guide to...
: Embarking on the Path to Impactful...
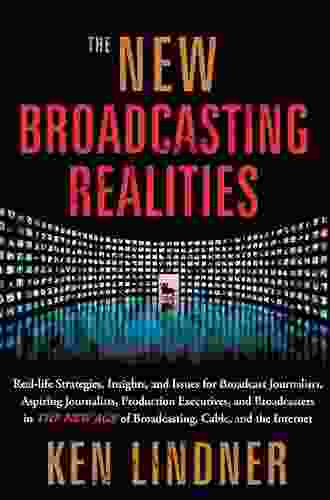

Unlock the Secrets of Captivating Radio Programming:...
In the fiercely competitive world of...
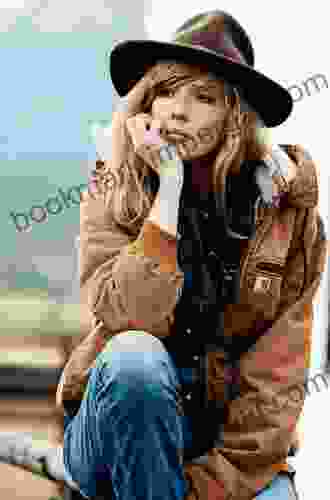

Unveiling the Enchanting World of Beth Inspired Eye...
A Realm of Imagination and Wonder Embark on...
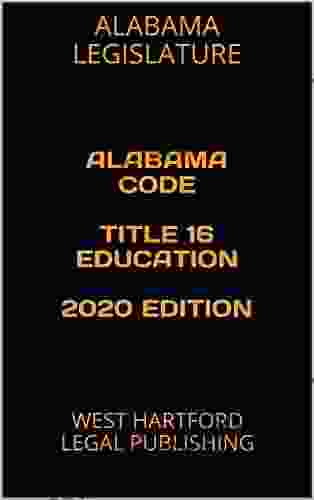

Unlock the Secrets of Legal Publishing with West Hartford...
West Hartford Legal Publishing, the renowned...
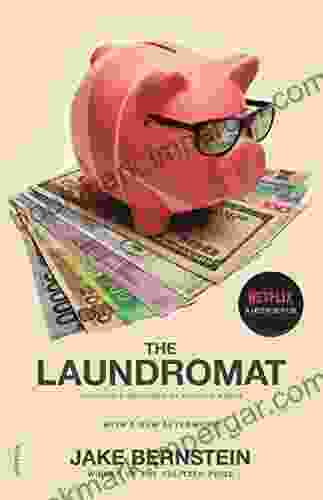

Unveiling the Secrets of the Panama Papers: Exposing...
The Panama Papers is a groundbreaking...
5 out of 5
Language | : | English |
File size | : | 14709 KB |
Text-to-Speech | : | Enabled |
Screen Reader | : | Supported |
Enhanced typesetting | : | Enabled |
Print length | : | 271 pages |
Lending | : | Enabled |